In 10 seconds? An artificial intelligence (AI) assistant can speed up the examination of breast cancer images. Using the tool, pathologists can detect dividing cells nearly 30% faster, raising the hope of better healthcare decisions for patients in the future.
What’s the breakthrough? Working with a group of 24 pathologists with different levels of experience, researchers found that 87.5% of them correctly identified more “mitotic figures” from microscopic images of breast cancer tissue when using an AI tool. What’s more, it reduced the average time to do so by 27.8%.
Mitotic figures? Try again without the techno-babble! A mitotic figure is just a cell that’s been caught in the middle of dividing (via a process called mitosis) at the time the breast cancer sample was taken. Pathologists can stain very thin slices of this tissue to more easily examine the structure and contents under a microscope, including to count how many cells are dividing in a particular sample. This is important because higher mitotic figure counts in addition to other “proliferation markers” (stuff that shows cells are growing) have been linked to poorer outcomes for breast cancer patients.
How did they test their AI tool? It’s complicated - the researchers used 320 breast cancer samples to train an algorithm to identify the mitotic figures. “L-view”- as it’s known - uses a “deep convolutional neural network”, which applies layers of filters to find useful landmarks in an image and identify dividing cells. It’s like going for an eye test and looking at letters on the wall as the optometrist applies different filters – your brain will pick out different bits of information in different scenarios in order to recognise the letters. The researchers tested their system with a group of reviewers, by asking them to quantify mitotic figures in multiple breast cancer images: both unaided and with the help of the AI assistant, which pre-marked potentially dividing cells. Incredibly, all but one of the reviewers demonstrated increased accuracy (87.5% ) when using the AI assistant. What’s more, the average time to spot them dropped by 10 seconds per image.
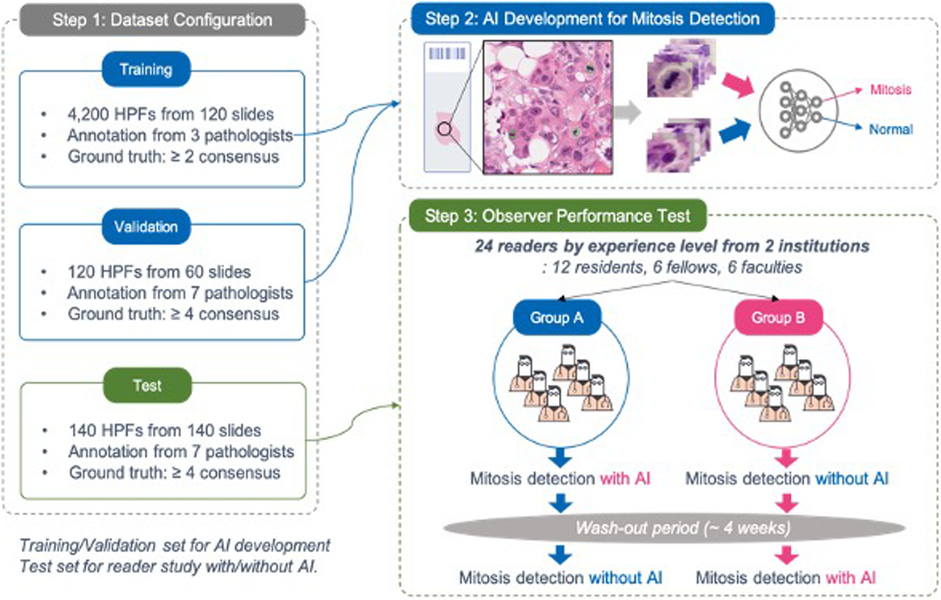
So how is this going to help people? The benefits of AI in breast cancer diagnosis have been demonstrated before, for example, when AI software beat human pathologists at detecting breast cancer from mammograms. However, this is the first time AI to count mitotic figures was tested with the pathologists in mind. As the authors put it, integrating a system like this could save pathologists around 16 minutes for every hour of counting, freeing them up for other tasks. With health systems around the world scrambling to recover from the COVID pandemic, time savers like this could be critical. What’s more, the improved accuracy of mitotic counting may one day lead to better outcomes for patients by aiding treatment decisions – fitting the right treatments to the right patients.
What happens next? There’s much more work to be done to get a technology such as this into daily clinical practice. The authors face problems in terms of large numbers of false negatives - a problem when trying to grade a tumour accurately. As ever in medicine, a large clinical trial would be needed with more samples and more reviewers to ascertain its true benefit to patients – and ensure safety. It’s more likely that a tool such as this would be integrated into a larger AI package to really nail down the “tumour grade” (to answer the question “how bad is the tumour and what’s the best strategy to treat it?”). Take this study for example, which uses genetic information and AI to distinguish early and late-stage breast cancer patients.
Anything I can do to help?
Yes! Citizen science is big in cancer research. One of the best things you can do to help AI in cancer projects like this one is to annotate images!
For example, The “Cell Slider” project funded by Cancer Research UK involved nearly 100,000 people to identify breast cancer cells from microscopic images, and they found that they could do it with high accuracy.
This is important, as one of the downfalls of AI is that it sometimes requires huge amounts of annotated images to train the algorithms properly.
Look out for similar projects in the future – you might help to improve the lives of people suffering from cancer!
Ed has distilled 8 research papers, saving you 28 hours of reading timeThis Digest has an evidence score of 3.8 out of 5
The Science Integrity Check of this 3-min Science Digest was performed by Flávia Oliveira Geraldes.